In Digital Transformation Technology is Not Everything
What matters most is how you go about implementing it.
To find out how to implement innovation that lasts, you can read about the latest trends, and advances in technology with our free e-book guides.
Explore the future of social housing repairs with this comprehensive guide to cutting-edge technology.
Streamline housing management with Propsys360's innovative, cloud-based solutions.
Discover the key to streamlining Service Charge Management
A new era of reliable, trusted and real-time housing technology on Propsys360
Navigate the digital landscape with confidence and set your business on a path to triumph in the digital era.
Explore Microservices: Optimise architecture, integrate seamlessly, and migrate effortlessly for the best-of-breed software solutions.
Begin Now! Harness Tech for Unstoppable Success. Dive into 'Become The Future You' for Future-Proof Triumphs.
Discover cost-cutting insights in our ebook - Reduce Cost of Digital Transformation. Unleash growth and savings in your business today!
Tackle the Digital Skills Gap head-on with our powerful ebook. Bridge the divide and future-proof your workforce now!
Explore the Benefits of Cloud Migration in our ebook. Embrace scalability, efficiency, and enhanced performance for your business.
Elevate Your Digital Strategy with Microsoft Technology Stack in our ebook. Unlock the potential of cutting-edge solutions and opportunities.
Navigate Disaster Recovery with ease using our comprehensive ebook. Protect your business from downtime and data loss effectively.
Speed up with Sprint Velocity. Gain insights into agile delivery and enhance efficiency in our ebook.
The Perfect Combination: DevOps and Agile Methodology. Learn how they drive innovation in our ebook.
Ensure Business Success with Digitalisation. Embrace digital transformation with our actionable ebook.
Uncover Benefits of DevOps Strategy. Drive collaboration and efficiency in our comprehensive ebook.
Overcome Common Challenges in Digital Business Transformation. Find solutions and thrive with our ebook.
Embrace Disruption for Growth. Discover how to stay ahead in the digital age in our eye-opening ebook.
Digital Future of Housing: Innovations Unleashed. Dive into the future of housing with our cutting-edge ebook.
Inspiration You Need
Our Clients
What Our Customers Have to Say
Notting Hill Genesis is on a complex digital transformation journey and needed a partner who could add
new agile global teams and bring in the latest technology solutions that the cloud offers.
The Neo Technology model allows us to scale our development capacity, accelerating our transformation programmes while future-proofing our business while achieving substantial industry cost savings.
Their integrated agile project teams deliver across a range of business requirements in partnership with our Product Owners, such as System Enhancements, Data Integration & Cloud Migration, enabling Notting Hill Genesis to accelerate our digital transformation goals.
The Neo Technology model allows us to scale our development capacity, accelerating our transformation programmes while future-proofing our business while achieving substantial industry cost savings.
Their integrated agile project teams deliver across a range of business requirements in partnership with our Product Owners, such as System Enhancements, Data Integration & Cloud Migration, enabling Notting Hill Genesis to accelerate our digital transformation goals.
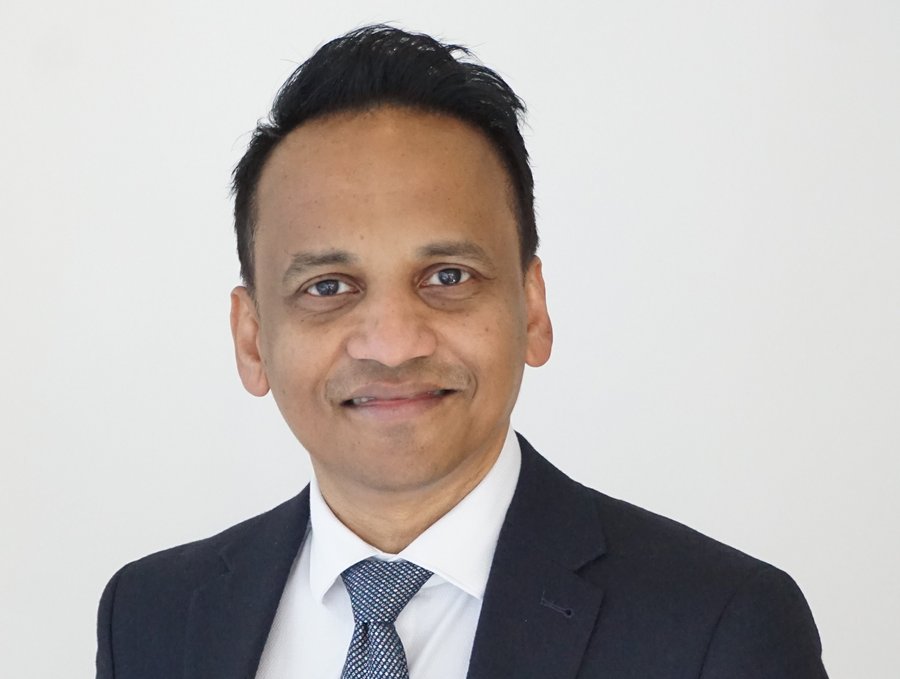
Neo Technology has helped Magnum Photos scale our operations, control our costs and improve processes whilst increasing system uptime to 100%.
In turn they helped us not only secure but also grow our revenue.
Thank you so much for acting as our true digital partner.
In turn they helped us not only secure but also grow our revenue.
Thank you so much for acting as our true digital partner.
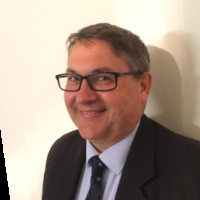
Neo Technology has helped us scale our operations globally.
We can now scale the business into multiple geo's while maintaining high-quality coding standards and turnaround times.
I would 100% recommend them.
We can now scale the business into multiple geo's while maintaining high-quality coding standards and turnaround times.
I would 100% recommend them.
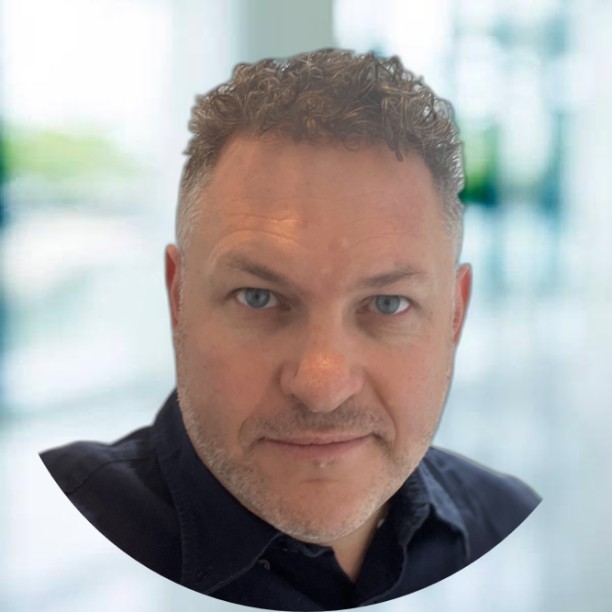
At VIVID Homes, we are proud to be the first adopters of the comprehensive Microsoft Dynamics Omnichannel solution in the UK, providing a centralised system for managing our customer communications.
In partnership with Neo Technology, we have enhanced this solution by leveraging the capabilities of Azure Integration Services. This integration enables seamless agent engagement and efficient work status sharing between the Omnichannel system and our SaaS-based work allocation platform.
The successful deployment of this solution has significantly increased transparency and improved the operational efficiency of our contact centre, allowing us to deliver greater value to our customers.
This achievement was made possible through the expertise and dedication of Neo Technology. We extend our sincere thanks to Neo Technology for their invaluable support.
In partnership with Neo Technology, we have enhanced this solution by leveraging the capabilities of Azure Integration Services. This integration enables seamless agent engagement and efficient work status sharing between the Omnichannel system and our SaaS-based work allocation platform.
The successful deployment of this solution has significantly increased transparency and improved the operational efficiency of our contact centre, allowing us to deliver greater value to our customers.
This achievement was made possible through the expertise and dedication of Neo Technology. We extend our sincere thanks to Neo Technology for their invaluable support.
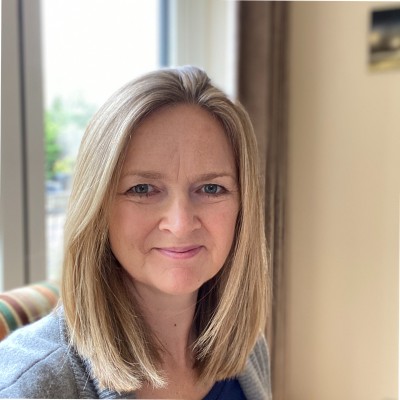